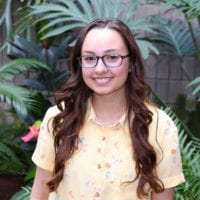
Automated Detection of Plant Venation
Plant grafting is an important agricultural technique because it allows for plants to become more resistant to disease, increase crop yield, or surpass juvenility. In grafting, the rootstock (roots) of one plant is attached to the scion (shoot) of another plant (Melnyk, 2015). A successful graft must undergo a healing process at the graft junction and join the vasculature of the rootstock and scion. If healed properly, water should be able to move from the roots, through the graft junction, and to the leaves. One way to test this is with fluorescent dye, like fluorescein, which allows for easy visualization of plant veins because it can track water movement. Our goal was to create a data set of fluorescent leaf vein images which will be used to make a recognition system, through machine learning, that can autonomously identify fluorescent veins. This recognition system will be utilized in plant grafting to provide more information on the healing process and success of a graft because it will be trained to know what healthy vasculature should look like. We believe that through fluorescent imaging and machine learning, plant vasculature can be visualized and understood in a more efficient way. To create this program, we selected plants based on venation pattern, presence of trichomes, waxiness of cuticle, distance of vein from leaf surface, and member of the nightshade family, which are commonly grafted plants. These leaves varied greatly, creating a large and diverse data set. To image the xylem, we introduced fluorescent dye, fluorescein or carboxyfluorescein (CFDA), to the roots. We noticed that parts of the images may pose issues in machine learning including autofluorescence, missing pieces, injury, or differences in the intensity of fluorescence. To account for these issues, hand annotations were created by tracing leaf veins, which was used help the computer recognize what a vein looks like. We concluded that fluorescein is better at imaging the xylem than CFDA, and we are still in the process of machine learning. We hope to continue building our data set and improving our recognition system in the future.
My Experience
My summer as a BTI intern has been such a rewarding experience and I cannot believe how much I learned in just ten weeks. Being able to work with my mentor, PI, graduate students, and peers, I have been able to fully experience what a research lab environment is like and learn new lab techniques and skills. My experience at BTI has given me more ideas about future opportunities that are available in graduate school, research, and careers. After this summer, I feel more prepared and confident to further my education in graduate school and work towards a Ph.D.