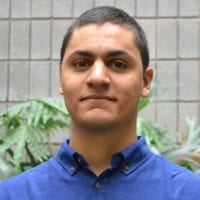
“Genome Wide Association Studies of Maize Leaf Area and Dry Weight”
Project Summary
Maize (Zea mays L.) is an economically important crop that accounts for approximately 90 million planted acres in the United States annually. Maize yields have octupled since the 1930s due to gradual acceptance of hybrid varieties, as well as breeding efforts aimed at optimizing canopy architecture for higher planting densities. Although leaf size has not been directly correlated with yield, it is an important characteristic of canopy architecture, especially as it relates to field-level transpiration and leaf area index. However, to our knowledge, very few attempts have been made to characterize the genetics underlying maize leaf area and dry weight.
To this aim, a subset of 465 maize inbred lines from the Wisconsin Diversity Set were grown in Maricopa, Arizona in 2017. At anthesis, leaves subtending the uppermost ear were harvested for imaging, drying and weighing. Subsequently, an image analysis workflow was developed in ImageJ to extract leaf area from the images. Best Linear Unbiased Predictions (BLUPs) for leaf area and dry weight were calculated and found to be strongly correlated (r2=0.91) with each other. [MAG1] By testing for associations between 177,000 genome-wide SNPs and these two traits, we identified significant association signals[MAG2] on chromosomes 3 and 5 for both traits. Closer investigation of these two signals when combined with RNA-seq data could reveal the underlying causative genes influencing the observed phenotype.
My Experience:
Learning that scientific experiments don’t always go exactly as planned helped me to grow as a scientist this summer. The experiment that we originally conducted was intended to reveal genes associated with maize leaf cuticle development, but my project eventually shifted its focus onto leaf size traits for which we had an abundance of data. Having tons of data from the get-go allowed me to practice data manipulation in RStudio which was an invaluable experience that I desperately needed for my future career. I wouldn’t yet consider myself an expert bioinformatician, but my skill level in the field has expanded dramatically since this internship began. I would like to thank BTI for administering this program and accepting me. I’d especially like to thank my mentor, Meng Lin, for helping me accomplish things of which I never thought myself capable. Thank you for lessening my fears of coding and statistics.