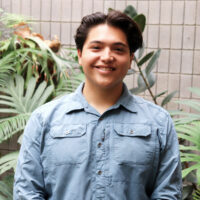
Predicting Rate of Recombination Events in the Maize Genome
In a world with a constantly changing climate, crops must be able to adapt to their environments and produce enough yield to sustain an ever-growing population. Plant breeders recognize this problem, but the process of taking ideal traits from a genetically diverse founder population, as well as removing deleterious, or unfavorable, traits from a population is inefficient due to meiotic recombination, which leaves unpredictability in the rate of which these events occur in crossover sites across the genome. Our hypothesis is that recombination rate, at both a local and global level, is predictable from a machine learning model using various chromatin and genomic features. The dataset we used to train a supervised machine learning model using a regression algorithm contains epigenetic and histone features (independent), most notably being methylation patterns and histone H3 variants, and the recombination rate (dependent) of chromosome 1 in maize. Using a local resolution (10kb) and a global resolution (100kb) to train and test the model to predict recombination rate on multiple genomic scales. In conclusion, we were able to predict the rate of recombination with a global accuracy of 56% and local accuracy of 50. The future direction of this project is aimed at improving the accuracy of our current model, through feature selection or dimension reduction. Then to adapt that model to use the DNA sequence alone as a predictor for recombination rate, allowing access to high accuracy recombination rate prediction with minimal hurdles in relation to sequencing.
My experience at BTI has been one involving much personal and professional growth. From meeting friends with similar interests and goals as mine, the weekly seminars and multitude of classes, to making professional relationships that have launched my understanding in bioinformatics, computational biology, and mainly machine learning in biology to beyond my expectations. My amazing mentor, Ruth Epstein, helped me understand the broader impacts all the way to the foundations of my project, while also showing me the daily work life of a Ph.D. student in the Pawlowski Lab. Overall I loved my summer internship at BTI and I believe that the best is yet to come, with my future goals being to join a Ph.D. program in computational biology.